Exploring Deepfakes: Are They Just Digital Trickery or a New Ethical Dilemma?
Imagine a world where seeing is no longer believing. Where the line between reality and fiction blurs to the point that distinguishing one from the other becomes a Herculean task. Welcome to the age of deepfakes, a technological marvel—and a potential menace—that's quietly reshaping our perception of truth. Have you ever watched a video of a famous politician saying something outrageous, only to discover it was a hyper-realistic fake? Or perhaps you've stumbled upon a clip of a deceased celebrity, seemingly brought back to life with uncanny precision. These are deepfakes, and they're more than just digital trickery—they're a harbinger of a new era in media, communication, and ethics.
As we stand at the precipice of this new reality, it's crucial to arm ourselves with knowledge. What are the mechanisms that power these convincing digital doppelgängers? How can they be used, for better or worse, across different sectors? And what does their emergence mean for the future of information integrity? In this blog post, we'll peel back the layers of deepfakes, examining not just their technical composition, but the broader implications they carry. Join us as we unravel the threads of this complex tapestry, exploring the fascinating and sometimes frightening world of deepfakes.
Understanding Deepfakes
A deepfake is an eerily accurate type of synthetic media where the likeness of a person in an existing image or video is replaced with someone else's, typically leveraging sophisticated artificial intelligence (AI) systems. The term "deepfake" itself is a blend of "deep learning" and "fake," indicative of the deep learning algorithms that drive the generation of these hyper-realistic manipulated videos and images. This technology relies on neural networks that analyze thousands of images or video frames, learning to mimic the appearance and mannerisms of individuals with alarming precision. As a result, deepfakes are becoming increasingly indistinguishable from genuine footage, raising concerns about their potential use in misinformation campaigns, identity theft, and other malicious activities.
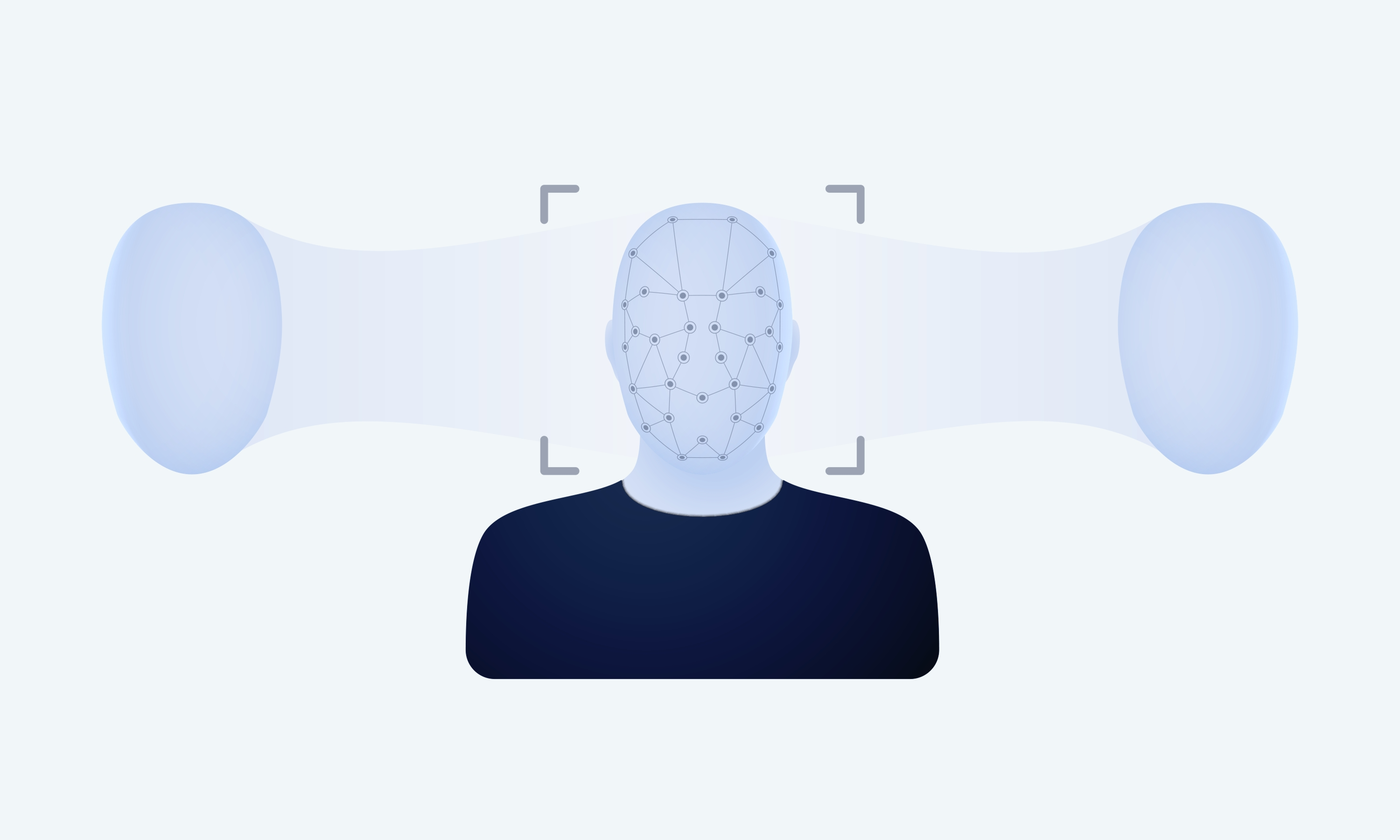
The creation of deepfakes extends beyond mere face-swapping; it involves simulating voice, facial expressions, and even body movements to create convincing forgeries. The implications of this technology are profound, as it challenges our perception of reality and truth in digital media. While there are benign applications, such as in filmmaking and entertainment, the potential for abuse cannot be overstated. With the rapid advancement of AI, the line between what's real and what's artificially generated is blurring, necessitating critical conversations about ethics, security, and the future of digital authenticity.
How Deepfakes Are Created
Deepfakes are generated using a type of AI called generative adversarial networks (GANs). In this process, two neural networks compete with each other: one generates images (the generator), while the other evaluates them (the discriminator), aiming to distinguish between the generated images and real images. Through this iterative process, the generated images become increasingly difficult to differentiate from authentic ones. In an era where AI-generated content can replicate human likeness with alarming precision, discerning truth from falsehood becomes a paramount concern. DeepBrain AI's deepfake detection technology stands as a bulwark against the tide of synthetic media, employing sophisticated algorithms to identify and neutralize potential threats.
DeepBrain AI's Deepfake Detection Technology
In an era where AI-generated content can replicate human likeness with alarming precision, discerning truth from falsehood becomes a paramount concern. DeepBrain AI's deepfake detection technology stands as a bulwark against the tide of synthetic media, employing sophisticated algorithms to identify and neutralize potential threats.
DeepBrain AI's solution is a comprehensive system designed to detect deepfakes in various forms, including video, image, and audio content. The technology aims to provide a real-time defense mechanism, ensuring that the authenticity of content is verifiable, thus maintaining the trust of viewers and consumers.
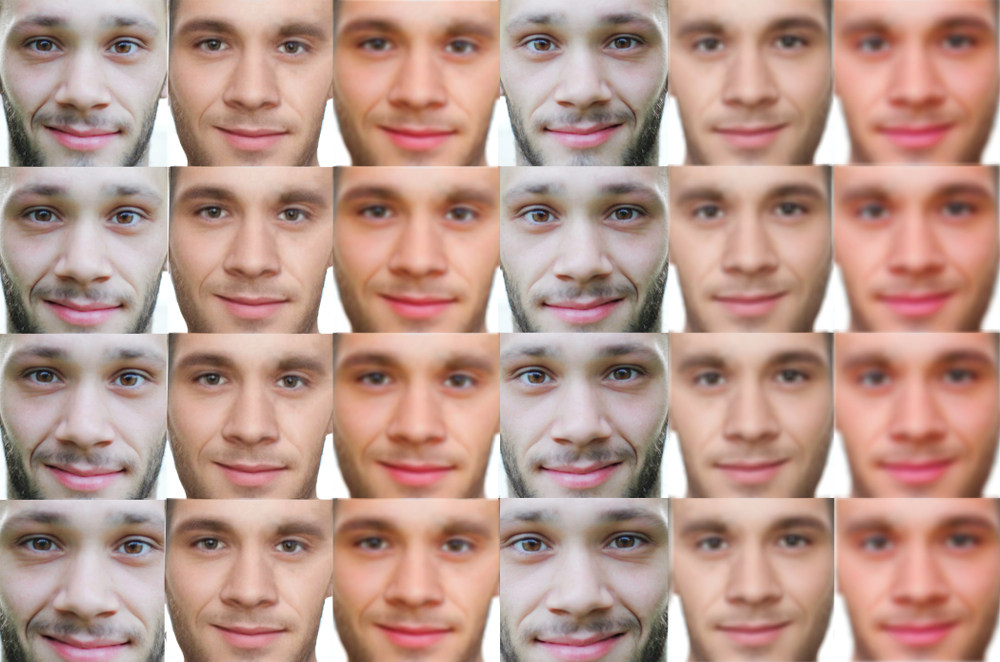
Deepfake Video Synthesis and Image Detection
DeepBrain AI's technology addresses the challenge of detecting deepfakes through multiple specialized models:
- Deepfake Video Synthesis Detection: This model scrutinizes video content for signs of manipulation, leveraging advanced neural networks to spot discrepancies imperceptible to the human eye.
- Deepfake Image Detection: Images, just like videos, are susceptible to deepfake technology. DeepBrain AI's solution can detect subtle manipulations, ensuring the integrity of still imagery.
- Deep Voice Detection: Audio deepfakes pose a unique threat by mimicking voices with high accuracy. DeepBrain AI's detection system analyzes vocal patterns and sound anomalies to identify these sophisticated forgeries.
.png)
Cutting-Edge Detection Models
DeepBrain AI's arsenal includes an array of models, each employing distinct methods to enhance the detection of deepfakes:
- EfficientNet + Vision Transformer: By combining the benefits of CNNs with Vision Transformers, this model offers a potent mix of speed and accuracy. It is trained on a comprehensive dataset, including DeepBrain AI's extensive collection of deepfake detection data, and utilizes augmentation techniques to improve generalization across varied data sets.
- Lip Forensics: Targeting the mouth movements in videos, this model capitalizes on the common issue of lip-sync errors in deepfakes. It uses the ResNet-18 architecture to extract mouth features and then applies the MS-TCN model to assess the video's authenticity.
- ICT Deepfake: Focusing on facial identity, this model uses face-swapped images for training, allowing it to recognize unique facial features without needing separate deepfake data. The transformer-based approach enables it to detect deepfakes regardless of the generation technique.
- GANDCT Analysis: This model operates on the premise that generative models leave identifiable patterns in the frequency domain during the image upscaling process. By converting images to the frequency domain using discrete cosine transform (DCT), it can pinpoint these patterns, which are visualized as heatmaps, to detect GAN-based image manipulations.
.jpg)
Incorporating DeepBrain AI's deepfake detection solutions allows organizations to stay ahead of fraudulent media's curve, ensuring the credibility of digital content and protecting against the malicious applications of deepfake technology.
.png)
Types of Deepfakes
There are generally two main types of deepfakes:
- Face Swaps: This involves replacing the face of one person with the face of another in a video or image. This type of deepfake is commonly seen in viral internet videos and has been used for both entertainment and malicious purposes.
%20(1).png)
2. Full Body Deepfakes: These are more complex and involve generating an entire person’s likeness, including their body movements and actions. This type of deepfake requires more data and processing power to create a believable result.
.png)
Capabilities of Deepfakes
Deepfakes can be incredibly realistic, making it difficult for humans and even some software to detect them. They are capable of:
- Mimicking facial expressions and movements
- Synthesizing realistic human voices
- Altering the context or content of a video or image
- Creating entirely new content that appears authentic
Use Cases of Deepfakes
Deepfakes have a variety of applications, both positive and negative:
Positive Applications
- Entertainment: In movies and video games, deepfakes can be used to create realistic characters or bring deceased actors back to life for cameo appearances.
- Education: Historical figures could be brought back to life to deliver lectures or participate in interactive learning experiences.
- Art: Artists are using deepfake technology to push the boundaries of creativity and explore new forms of expression.
%20(1).png)
Negative Applications
- Misinformation: Deepfakes can be used to create fake news or manipulate public opinion by portraying individuals saying or doing things they never did.
- Fraud: There's potential for deepfakes to be used in scams, such as creating fake video evidence or impersonating individuals for financial gain.
- Harassment: Deepfakes can be used to create non-consensual pornography or to harass and blackmail individuals.
%20(1).png)
Misconceptions and Concerns
Common Misconceptions
- Detectability: While deepfakes can be convincing, there are often subtle clues that can give them away, such as unnatural blinking or inconsistent lighting.
- Ease of Creation: Creating a high-quality deepfake requires significant technical skill, computing power, and data, although user-friendly deepfake tools are becoming more accessible.
Concerns
- Ethics: The potential for deepfakes to be used unethically is a major concern, particularly in spreading disinformation and violating consent.
- Legal: There is an ongoing debate about the legal implications of deepfakes and how existing laws apply to their creation and distribution.
- Security: Deepfakes pose security risks, including the potential to bypass facial recognition systems or create convincing fake identities.
How Can We Balance the Innovative and Risky Aspects of Deepfakes?
Deepfakes represent a fascinating yet concerning advancement in AI technology. While they offer exciting opportunities in creative and educational fields, they also raise significant ethical and security questions. As this technology continues to evolve, it is crucial for individuals, organizations, and governments to understand deepfakes and work towards solutions that prevent their misuse while harnessing their potential for positive impact.
In navigating the world of deepfakes, staying informed and vigilant is key. Whether you're a content creator, a consumer of media, or simply an interested observer, being aware of the capabilities and risks of deepfakes is essential in the modern digital landscape.